Towards an improved methodology for automated readability prediction
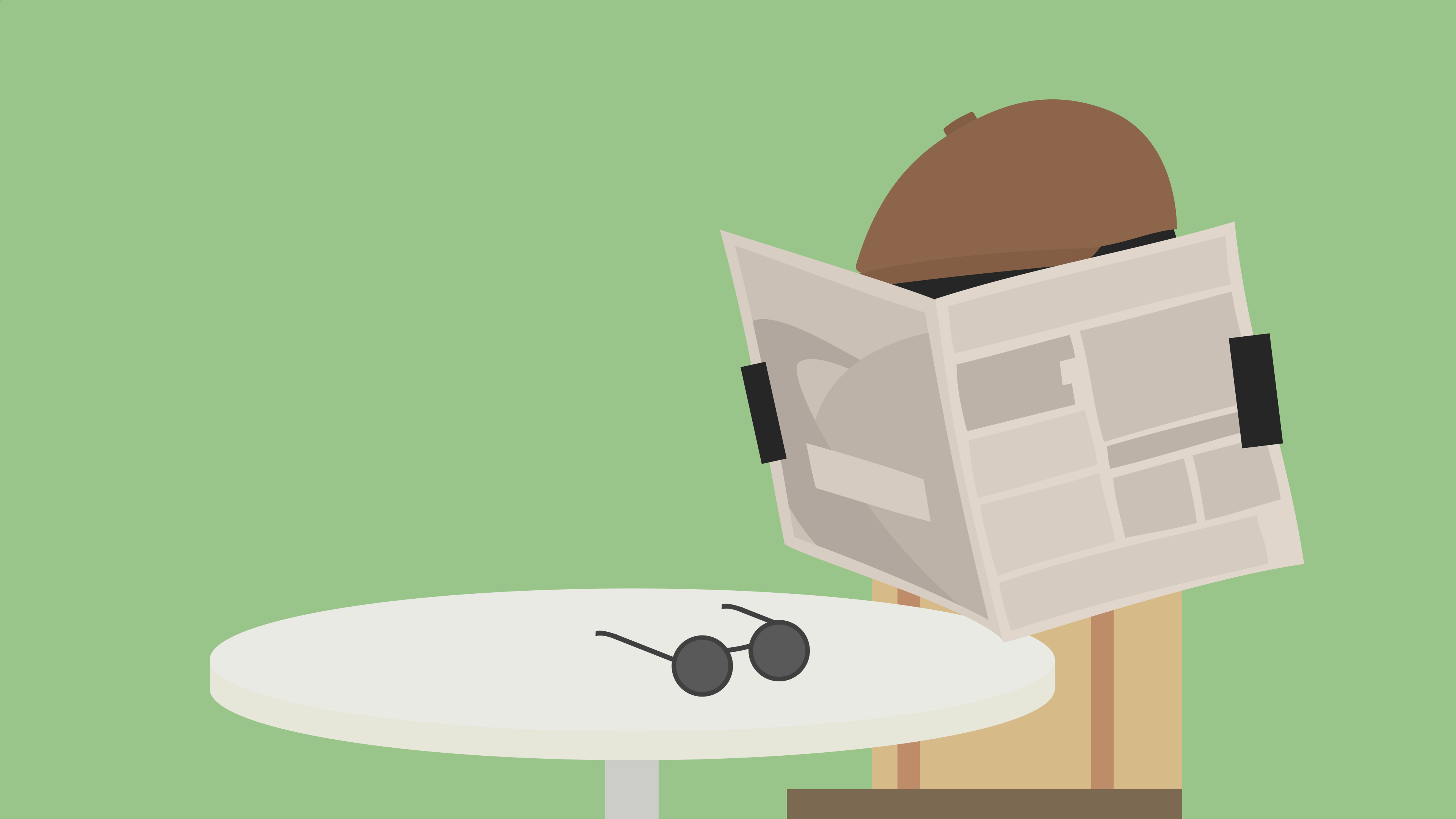
What exactly makes one text more “readable” than another? There are various ways to define “readability” and all of them can be correct. Some people define it as the level of proficiency that readers need to understand a text, while for others it is also important that a text is inviting to readers.
This week’s paper looks at the concept of readability from that first point of view, as calculated using commonly used readability formulas.
Readability formulas are mathematical formulas that consist of a number of text characteristics (variables) and some constant weights. Common examples of text characteristics include average word length, sentence length, word variety, and ratio of commonly occurring words.
These formulas are used to determine the of texts for pupils and students among others.
The researchers took a bunch of different, commonly used readability formulas for Dutch, Swedish, and English:
-
Cito leesbaarheidsindex voor het basisonderwijs (Dutch)
-
Cito leesindex technisch lezen (Dutch)
-
Flesch-Douma (Dutch)
-
Leesindex Brouwer (Dutch)
-
Läsbarhetsindex Björnsson (Swedish)
-
Flesch Reading Ease (English)
-
Dale-Chall Reading Grade Score (English)
-
Coleman-Liau Index (English)
-
Flesch-Kincaid Grade Level (English)
-
Gunning Fog Index (English)
-
ARI: Automated Readability Index (English)
-
SMOG: Simple Measure of Gobbledygook (English)
These formulas were applied to texts from four different corpora in two different languages (Dutch and English):
-
Eindhoven Corpus (Dutch)
-
SoNaR (Dutch)
-
British National Corpus (UK English)
-
Penn Treebank (US English)
Correlations were computed between the readability formulas, variables within those formulas, and text characteristics for each corpus.
Like I said, a lot of findings based on correlations.
Many readability formulas are based on some notion of word length, e.g. the average number of characters, syllables, or words with more than x characters or syllables. It should not come as a surprised that all these different ways to measure word length are strongly correlated with each other.
The same can be said about the various methods that can be used to compute sentence lengths.
Finally, the type/token ratio is a metric that describes the number of unique words divided by the total number of words. This is a rather unique metric that isn’t related to any of the other metrics that are described in this paper.
In general, readability scores are moderately to strongly correlated with word length: texts with longer words are thought to be more difficult to read, regardless of the precise method used to measure word length.
Interestingly, included in the study seem to suggest that texts with shorter words are actually harder to read, which is clearly the opposite of what we would expect!
Even though readability formulas tend to be tweaked for specific languages, the results show that formulas for English and Swedish can also be safely used for Dutch. This is likely due to the fact that readability formulas tend to be based on language-independent properties like word and sentence lengths.
Having said that, there are a few metrics that are language-specific, e.g. metrics based on lists of commonly occurring words in a language. One would expect that those would work better for their corresponding languages, but that doesn’t seem to be entirely true.
And of course there are also some minor differences between languages that ideally should be taken into account. For instance, compounds in Dutch are generally written as one word (e.g. “webdeveloper” rather than “web developer”). This increases the likelihood that words look long, without actually increasing the difficulty of the word.
As I already wrote above, many of the text characteristics are somewhat related to each other. Principal component analysis can be used to determine the number of independent text characteristics.
It turns out that a single latent factor can already explain almost all of the variance between the different formulas. In other words: they’re basically interchangeable!
Finally, the researchers performed collinearity tests to determine whether the methodology used to construct the readability formulas is valid. Such tests yield so-called condition numbers. High condition numbers are undesirable, as it suggests that collinearity between variables may be harmful.
Many of the computed condition numbers for the readability formulas are quite high. Some are even so high that the formulas probably shouldn’t be used for texts in the corpora!
-
Most readability formulas are based on language-independent text characteristics, like word and sentence length
-
Most readability formulas measure roughly the same thing and are conceptually virtually interchangeable
-
However, due to collinearity not all readability formulas are always suitable for your texts!