Fake news vs satire: A dataset and analysis
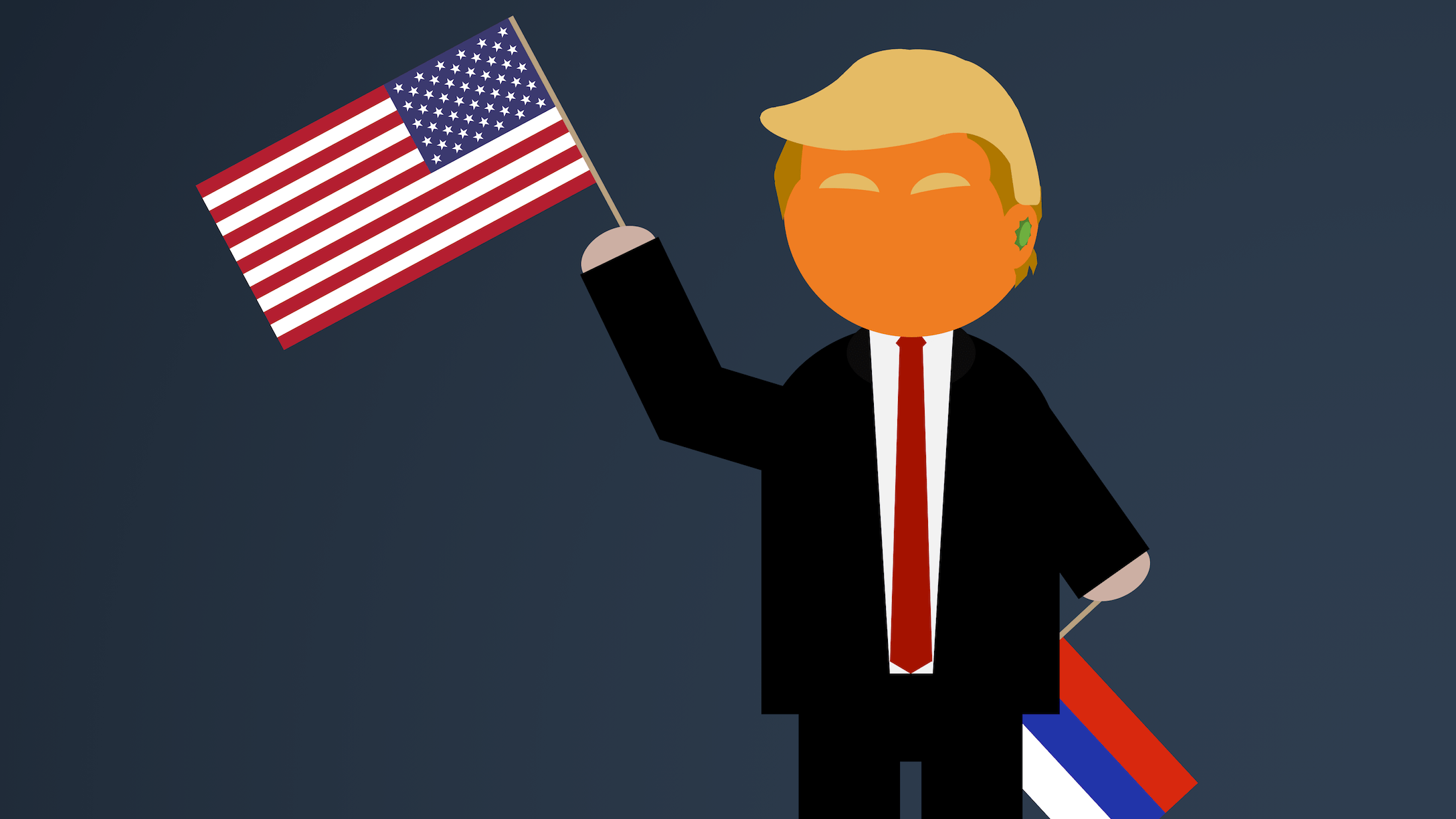
Social media platforms have a moral obligation to prevent the spread of fake news, while still allowing users to freely share satire. This can only be achieved using advanced content filters. Golbeck et (many, many) al. compiled a dataset of fake news and satire, and built a simple classifier that can tell the two types of stories apart.
While fake news isn’t a completely new phenomenon, its impact on society at large has increased significantly in the past few years. This is partially due to social media like Facebook, Twitter, and YouTube, which facilitate the spread of fake news.
To combat fake news at web scale, we need a way to automatically distinguish fake news from other types of information.
This is not an easy task. Let’s first look at the definition of fake news:
Fake news is information, presented as a news story that is factually incorrect and designed to deceive the consumer into believing it is true.
Now let’s look at things that are not fake news:
-
Satire presents information as news and contains false information, but isn’t actually intended to deceive;
-
Legitimate news stories may unintentionally contain factual errors;
-
Legitimate, factually correct news stories that cover a topic that’s disliked by certain parties and therefore labelled as “fake news”.
Because we only want to prevent the spread of real fake news, an automated classifier must be able to tell fake news and satirical articles apart.
The authors first created . Articles are selected from many different sources to reduce the chance that topics discussed in the articles or a particular publication’s writing style would affect the classifier. Articles that are not (x)or satire are excluded from the dataset for a similar reason.
To find differences in language (the vocabulary that’s used), the authors represented each article as a simple word vector that’s labelled either “Fake” or “Satire”. A model was trained using a multinomial naive Bayes classifier and tested using 10-fold cross validation.
Then, the authors created a list of themes that appeared throughout the dataset:
-
Hyperbolic positions against one person or group;
-
Hyperbolic position in favour of one person or group;
-
Discrediting a normally credible source;
-
Sensationalist crimes and violence;
-
Racist messaging;
-
Paranormal theories; and
-
Conspiracy theories.
After manually labelling each article in the dataset using these themes, the authors performed an analysis of the correlations between them.
The multinomial naive Bayes classifier manages to achieve an accuracy of 79.1%, which suggests that there are clear differences in the language used between fake news and satire.
More than two-thirds of all articles take hyperbolic positions against a person, while conspiracy theories appear in almost 30% of all articles, of which most are fake news stories. Sensationalist crimes are another theme that appears more often in fake news than in satire. Paranormal themes on the other hand, are more common in satire.
Fake news stories tend to have more themes than satirical articles. The overall most common pairing of themes was formed by hyperbolic criticism and conspiracy theories, e.g. articles about President Obama’s birth certificate.
When the authors subsequently added themes to the word vectors, they discovered that the word vectors can also be used to determine the presence of certain themes in an article.
-
A simple bag of words approach is enough to tell fake news and satirical articles apart
-
Hyperbolic criticism is a popular theme in both fake news and satire
-
Fake news typically has different combinations of themes than satirical articles